AI Ethics and Values: A Complete Guide to Ethical Principles
.png)
Overview: Why AI Ethics Matters
As artificial intelligence (AI) continues to reshape industries and redefine societal norms, the ethical considerations surrounding its development and deployment have become more critical than ever. The potential of AI to drive innovation and efficiency is vast, but so are the risks associated with its misuse or unintended consequences. Issues such as algorithmic bias, lack of transparency, and accountability gaps have highlighted the urgent need for a structured approach to ethical AI governance.
Adopting robust ethical principles ensures that AI systems are not only innovative but also aligned with societal values, regulatory frameworks, and global standards. The following outlines the core ethical principles of AI, providing actionable insights and best practices for organisations aiming to build AI systems that uphold the highest standards of responsibility and integrity.
What is AI Ethics?
AI ethics refers to the principles and practices that guide the development, deployment, and governance of AI systems. These principles aim to ensure AI serves humanity while minimizing harm, promoting fairness, and upholding human rights.
Why it’s important:
- Avoids unintended consequences like biased outcomes or discriminatory practices.
- Builds trust and accountability among stakeholders, including users, regulators, and developers.
- Supports compliance with legal and regulatory frameworks, safeguarding organisations from penalties.
The Core Ethical Principles in AI
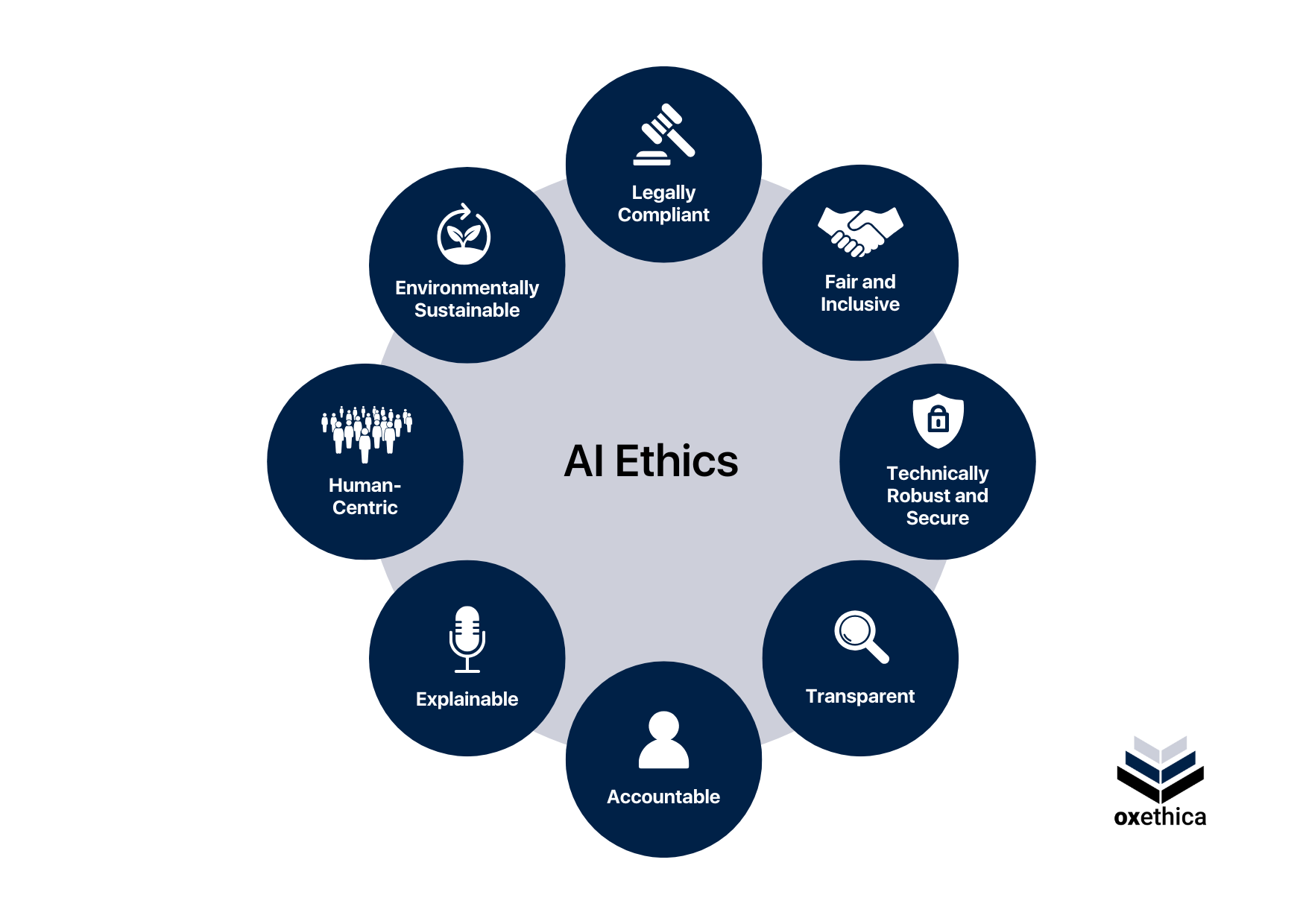
1. Legally Compliant
Definition:
Legally compliant AI aligns with regional and global regulations, ensuring adherence to laws governing data privacy, intellectual property, and human rights.
Best Practices:
- Conduct regular compliance audits throughout the AI lifecycle.
- Ensure partners and collaborators adhere to legal requirements.
Examples:
- Compliance with the EU AI Act to protect user rights.
- Aligning with data privacy laws like GDPR.
2. Fair and Inclusive
Definition:
Fair and inclusive AI ensures non-discrimination and respects diversity, addressing issues like bias and unequal access to technology.
Best Practices:
- Assemble diverse AI development teams.
- Use datasets that are balanced, representative, and consent-driven.
- Rigorously test for bias at all stages of the AI lifecycle.
Examples:
- AI recruitment tools that avoid gender or racial bias.
- Chatbots designed for multilingual accessibility.
3. Technically Robust and Secure
Definition:
Robust AI is designed to function reliably and securely, even under adverse conditions, ensuring minimal risk of harm.
Best Practices:
- Implement safeguards like fail-safes and redundancy.
- Use adversarial training to address vulnerabilities.
- Adopt robust risk management frameworks.
Examples:
- Autonomous vehicles with built-in fail-safe mechanisms.
- AI-powered fraud detection systems with multi-layered security.
4. Transparent
Definition:
Transparency in AI ensures users understand an AI system’s purpose, capabilities, and limitations.
Best Practices:
- Disclose the AI’s purpose and data usage.
- Provide detailed documentation on AI logic and decision-making.
Examples:
- Transparent AI-powered recommendation systems for e-commerce.
- AI ethics dashboards for end-users and regulators.
5. Accountable
Definition:
Accountable AI places responsibility on developers and providers for AI outputs, ensuring ethical governance and explainability.
Best Practices:
- Conduct regular assessments and monitoring.
- Assign clear roles and responsibilities to avoid accountability gaps.
Examples:
- Traceable decision logs for AI-driven credit scoring systems.
- Ethical oversight boards in AI development companies.
6. Explainable
Definition:
Explainable AI breaks down its decision-making process, promoting trust and enabling oversight.
Best Practices:
- Perform technical audits to validate AI decision-making.
- Develop user-friendly reports detailing AI predictions and logic.
Examples:
- AI diagnostic tools in healthcare with explainable outcomes.
- Explainable AI in autonomous drone operations for military purposes.
7. Human-Centric
Definition:
Human-centric AI prioritizes human goals and well-being, enhancing capabilities rather than replacing them.
Best Practices:
- Incorporate human oversight in critical decision-making.
- Develop systems that enhance user well-being.
Examples:
- AI assistants designed to aid productivity without compromising privacy.
- Educational AI tools that empower marginalized communities.
8. Environmentally Sustainable
Definition:
Environmentally sustainable AI minimizes its ecological footprint while contributing to climate action.
Best Practices:
- Use energy-efficient AI models powered by renewable energy.
- Develop AI solutions that promote sustainability and circular economy practices.
Examples:
- AI models for optimizing renewable energy grids.
- Machine learning tools for climate change research.
Best Practices for Implementing AI Ethics
Implementing AI ethics is essential for building systems that are trustworthy, fair, and aligned with societal values. Below are four key practices to guide ethical AI development, tailored to ensure organisations can navigate this complex landscape effectively:
1. Conduct Ethical Impact Assessments Regularly
Organisations must evaluate the potential societal, environmental, and individual impacts of AI systems throughout their lifecycle. Ethical impact assessments (EIAs) help identify risks like bias, privacy concerns, and unintended outcomes. These assessments should be an ongoing process, adapting as AI systems evolve.
2. Build Transparency and Explainability into Systems
Transparency ensures stakeholders understand how AI systems function, their limitations, and potential biases. Explainability mechanisms should be integrated to provide clear insights into decision-making processes, enhancing trust and accountability. Detailed documentation and open communication with users are critical.
3. Promote Diversity and Inclusivity in AI Development
Diverse teams lead to more inclusive AI systems. By including a variety of perspectives—spanning gender, ethnicity, cultural backgrounds, and expertise—organisations can reduce the risk of biased or discriminatory outcomes. Inclusivity also fosters innovation and enhances the real-world applicability of AI solutions.
4. Leverage AI Governance Platforms
AI governance platforms, such as oxethica, provide organisations with the tools and frameworks needed to monitor, evaluate, and align AI systems with ethical principles. Oxethica empowers teams to ensure compliance with global standards, enhance accountability, and implement trustworthy AI governance practices across the development lifecycle.
5. Align with Global Standards for Ethical AI
Following recognized guidelines, such as those from OECD and UNESCO, ensures organisations meet international expectations for fairness, transparency, and responsibility. These standards provide a reliable foundation for building ethical AI systems while minimizing regulatory and societal risks.
Conclusion: Building a Responsible AI Future
Adopting ethical principles in AI development is not just a regulatory requirement but a moral obligation. By prioritizing fairness, transparency, and accountability, organisations can build systems that are not only innovative but also trustworthy and beneficial for society. AI ethics isn’t just a technical challenge—it’s a shared responsibility to ensure technology serves humanity’s best interests.
More on AI regulation
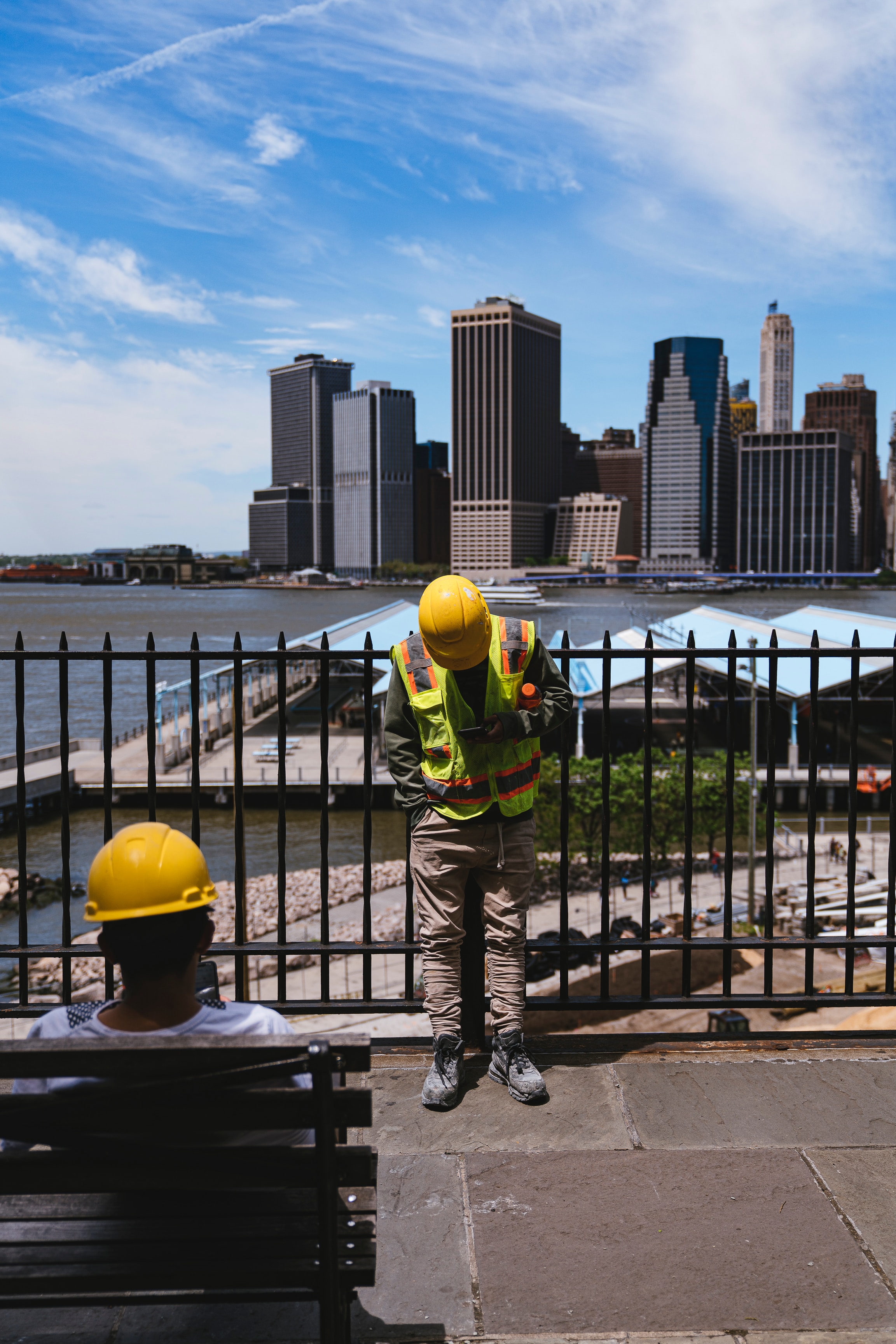